Multi-modal AI
Multi-modal AI refers to systems integrating and processing data from multiple modalities—text, images, audio, and video—to provide more prosperous and contextualized insights. Unlike traditional AI models specializing in one data type, multi-modal systems can understand the interplay between different data sources, enabling more robust decision-making and improved accuracy. These systems leverage deep learning architectures that fuse diverse inputs, resulting in better applications that capture the complexities of real-world scenarios.
At the heart of multi-modal AI is the ability to learn joint representations. For example, a system might combine visual cues from images with descriptive text to generate more accurate content summaries or to perform enhanced object recognition. This integration supports applications in healthcare (such as medical imaging combined with patient records), autonomous driving (integrating video feeds with sensor data), and digital media (merging audio and visual content for improved sentiment analysis).
A key benefit of multi-modal AI is its versatility :
- Enhanced Decision-Making – AI processes multiple data streams for better accuracy.
- Improved User Experience – More natural and interactive AI systems.
- Advanced Real-Time Processing – Enables AI to interpret complex real-world scenarios.
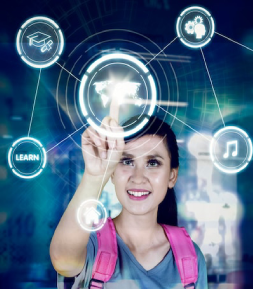
Use Cases :
1- Healthcare Diagnostics: Combining MRI scans with electronic health records (EHRs) to improve disease diagnosis and treatment recommendations.
2- Autonomous Vehicles: Merging LIDAR, camera, and radar data to enhance object detection, obstacle avoidance, and decision-making in real-time.
3- Digital Media & Marketing: Integrating social media text, images, and video content to analyze consumer sentiment and predict trends.
4- Security & Surveillance: Fusing audio and video feeds for enhanced monitoring and anomaly detection, such as in public safety applications.
Multi-modal AI opens doors to innovations and helps organizations tackle complex challenges where multiple data sources must be considered simultaneously. As industries continue to digitize, the need for systems that can understand and correlate diverse inputs will grow, making multi-modal AI an essential component of future innovative applications.